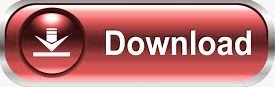
Read moreĬaravan Insurance Customer Profile Modeling with R Conversely, if ε is large enough that the tube can enclose all the data, the error becomes zero, there is no tradeoff to make, and the algorithm outputs the flattest tube that encloses the data irrespective of the value of C. In the degenerate case ε=0 the algorithm simply performs least-absolute-error regression under the coefficient size constraint, and all training instances become support vectors. The larger C is, the more closely the function can fit the data. The upper limit restricts the influence of the support vectors on the shape of the regression function and is a parameter that the user must specify in addition to ε. This tradeoff is controlled by enforcing an upper limit C on the absolute value of the coefficients α i. 7.3C there is no tube with error 0, and a tradeoff is struck between the prediction error and the tube’s flatness. 7.3A and B, where there is a tube that encloses all the training data, the algorithm simply outputs the flattest tube that does so. We have mentioned that as well as minimizing the error, the algorithm simultaneously tries to maximize the flatness of the regression function. In contrast to the classification case, the α i may be negative. As with classification, all other points have coefficient 0 and can be deleted from the training data without changing the outcome of the learning process-just as in the classification case, we obtain a so-called sparse model. The support vectors are all those points that do not fall strictly within the tube-i.e., the points outside the tube and on its border. Īs with classification, the dot product can be replaced by a kernel function for nonlinear problems. X = b + ∑ i is support vector α i a ( i ) ⋅ a. (There are, however, versions of the algorithm that use the squared error instead.) Another difference is that what is minimized is normally the predictions’ absolute error instead of the squared error used in linear regression. Also, when minimizing the error, the risk of overfitting is reduced by simultaneously trying to maximize the flatness of the function. The crucial difference is that all deviations up to a user-specified parameter ε are simply discarded. As with regular support vector machines, we will describe the concepts involved but do not attempt to describe the algorithms that actually perform the work.Īs with linear regression, covered in Section 4.6, the basic idea is to find a function that approximates the training points well by minimizing the prediction error. However, support vector machine algorithms have been developed for numeric prediction that share many of the properties encountered in the classification case: they produce a model that can usually be expressed in terms of a few support vectors and can be applied to nonlinear problems using kernel functions. The concept of a maximum margin hyperplane only applies to classification. Pal, in Data Mining (Fourth Edition), 2017 Support Vector Regression y =ax + b is the equation of a line and is a very simple example of a hyperplane.Extending instance-based and linear models For a three dimensional space, a line will be considered as a hyperplane. Hyperplane is a subspace having one dimension less than the space under consideration. This type of data where a linear hyperplane can classify the data into the required categories, is a linearly separable dataset.Īim of the SVM is to find the optimal hyperplane that is capable of separating the corresponding plane. Therefore, two of the soap can be segregated based on the decision boundary of 135 gm. The organic soap is having weight greater that 135 gm and the other one has weight smaller than it. The manager observed a trend in the weight of two soaps. In past days, they have observed that a few of the customers are getting a normal soap instead of organic soap. Lets consider a case study, a factory produces two types of soaps: organic soap and normal soap. We will understand linear separability through some examples.
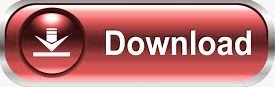